Feasibility and Acceptability of Using a Telehealth Platform to Monitor Cardiovascular Risk Factors
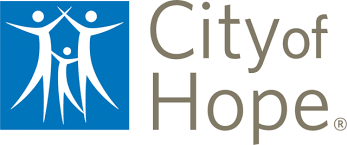
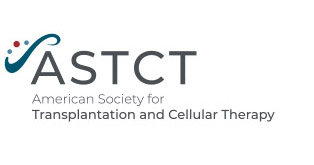
Feasibility and Acceptability of Using a Telehealth Platform to Monitor Cardiovascular Risk Factors in Hematopoietic Cell Transplantation Survivors at Risk for Cardiovascular Disease
1Department of Hematology and Oncology, Children’s Hospital Los Angeles, Los Angeles, California
2Department of Population Sciences, City of Hope, Duarte, California
3Perelman School of Medicine at the University of Pennsylvania, Philadelphia, Pennsylvania
4Department of Hematology and Oncology, Seattle Children’s Hospital, Seattle, Washington
5Department of Hematology and Hematopoietic Cell Transplantation, City of Hope, Duarte, California
ABSTRACT
Cardiovascular disease (CVD) is a leading cause of morbidity and mortality in hematopoietic cell transplantation (HCT) survivors. In these patients, such risk factors as hypertension, diabetes, obesity, and physical inactivity are important modifiers of CVD risk. However, the period when HCT survivors are at greatest risk of developing these risk factors, and in turn CVD, coincides with a drop in engagement in survivorship care. We examined the feasibility and acceptability of a 4-week remote risk-based monitoring (blood pressure monitor, weight scale, pulse oximeter, glucometer) and management program in 18 (11 allogeneic and 7 autologous) HCT survivors at intermediate-high risk of CVD. The median patient age was 66 years (range, 53 to 74 years), 67% had hypertension, 22% had diabetes, 11% were obese (body mass index ≥30 kg/m2), 56% were at intermediate risk of CVD, and 44% were at high risk of CVD. Weekly compliance with the remote monitoring schedule (≥3 readings/week using all devices) ranged from 72% in week 1 to 83% in weeks 2 to 4. Fifteen participants (83%) generated 86 alerts that were outside the predetermined range of normal; 63 of these readings (73%) normalized without intervention, and 23 (27%) necessitated triage by the study research nurse. Nearly all participants reported that the study kept them motivated and involved in their healthcare, and >85% agreed that the study supported their healthcare goals, helped them learn and manage their health conditions, and increased their access to healthcare. These findings may set the foundation for innovative risk-based and remote interventions to reduce the burden of CVD in this growing population of patients.
Key Words
Late effects, Hematopoietic cell transplantation, Cardiovascular disease, Cardiovascular risk factors, Survivorship, Telehealth, Telemedicine, Remote patient monitoring, mTelehealth, mHealth, eHealth
INTRODUCTION
An estimated 160,000 hematopoietic cell transplantation (HCT) survivors are living in the United States today, and that number is expected to exceed 500,000 by the year 2030 [1,2]. In these survivors, cardiovascular diseases (CVDs), such as coronary artery disease and heart failure, are a leading cause of morbidity and mortality, often occurring earlier than would be expected for the general population [3,4]. Outcome following onset of CVD in HCT survivors is poor; 5-year survival is <50% [4], emphasizing the need for innovative prevention strategies that address the changing burden of CVD risk over time.
There are currently no established evidence-based targeted interventions to reduce CVD risk in long-term HCT survivors, due in part to a paucity of information on appropriate risk stratification for targeted interventions after HCT. We recently developed and validated a risk prediction model to stratify 1-year HCT survivors into low-, intermediate-, and high-risk groups, corresponding to a 10-year cumulative incidence of CVD of 3.7%, 9.9%, and 26.2%, respectively [5]. In these patients, hypertension, diabetes, obesity, and lack of physical activity were all important modifiable risk factors for CVD [6]. This model’s 1-year post-HCT starting time point capitalized on the so-called “teachable moment” effect [7], whereby survivors, having survived 1 life-threatening disease, may be more motivated to try and prevent additional illness. However, studies in long-term HCT survivors indicate that the period when HCT survivors are at greatest risk of developing modifiable CV risk factors, and in turn CVD, often coincides with a drop in engagement in cancer-center directed care [8]. In this context, new models of care are needed to address the barriers and gaps in survivorship care.
The past decade has seen a burgeoning interest in telemedicine as a means to improve the management of such health conditions as diabetes mellitus, heart failure, and chronic lung disease 9, 10, 11. Telemedicine aims to fundamentally change the social contract between patients and healthcare providers in hopes of providing a greater focus on prevention, monitoring of chronic conditions, and patient-centered care. Remote patient monitoring (RPM) is a subcategory of telemedicine that allows patients to use mobile devices to upload health data and send it to a healthcare provider. RPM can facilitate simpler communication, support patient self-management, and improve the overall quality of care and patient outcomes with lower healthcare costs than traditional models of in-person care [12]. There is a paucity of information on RPM in survivors of HCT. Accordingly, we sought to determine the feasibility and acceptability of RPM for the management of selected modifiable risk factors (ie, hypertension, diabetes, obesity, and physical inactivity) in HCT survivors at intermediate/high risk of CVD.
METHODS
Study Participants
Participants were identified from an existing electronic database of HCT survivors at City of Hope. Eligibility criteria included (1) 1-year post-HCT (±3 months) and in remission, (2) age ≥21 years at the time of enrollment, (3) intermediate or high CVD risk (10-year cumulative incidence of CVD of 9.9% to 26.2%) [5], and (4) ability to read and communicate in English. Survivors with clinically evident CVD (ie, coronary artery disease or cardiomyopathy under active medical management) or with uncontrolled hypertension (systolic blood pressure [BP] ≥180 mmHg and/or diastolic BP ≥120 mmHg) were ineligible. Self-reported questionnaires and electronic medical records were used to obtain data on demographics, medical history, and participant satisfaction with the RPM platform. The City of Hope Institutional Review Board approved this study, and written informed consent was obtained from all participants before the initiation of study procedures.
Study Procedures
All study participants received the mTelehealth (www.mTelehealth.com) Remote Patient Monitoring (RPM) Kit (mTelehealth, Delray Beach, FL), which included the following Bluetooth-enabled devices: BP monitor, weight scale, pulse oximeter, glucometer (for patients with diabetes mellitus), and a 4G-enabled Pod that allows transfer of data from the Bluetooth-enabled devices to the mTelehealth Patient Portal (Figure 1). Participants also received a Fitbit Charge 3 (Fitbit, San Francisco, CA) to track their daily steps. This third-party device was chosen for its reported compatibility with the mTelehealth Patient Portal. Study personnel met with all study participants at baseline, emphasizing the importance of routine monitoring of modifiable CV risk factors. They then trained the participants on how to obtain correct measurements, provided them with written instructions and illustrations (eg, correct placement of BP cuff on the arm), and entered their demographic and medical information into the patient portal. Participants were asked to perform all potentially eligible study measurements once daily, 3 times a week for a duration of 1 month. Participants not performing the minimum required study measurements received a reminder follow-up phone call. Readings were wirelessly transmitted to a secure HIPPA compliant website that could be accessed by the participant, study staff, or nurse.

To ensure participant safety, readings that exceeded pre-established thresholds (eg, systolic BP >160 mmHg, diastolic BP >100 mmHg, or heart rate >110 bpm; Supplemental Figures 1-5) triggered alerts. Alerts were monitored by a nurse at Reconnect4Health (R4H), a health-management company with expertise in the interpretation and validation of remote patient-reported data. On receipt of an alert, the nurse reviewed the participant’s data on the secure website and took various actions, including validating the alert, conducting a symptom check, asking participants to retake the reading, and providing participant-directed interventions (eg, reminder to take medication), escalating to calls to physicians and/or emergency care if appropriate responses had not been met (Figure 1). The follow-up calls were completed Monday through Friday, 9 am to 5 pm EST. After receiving an alert, the nurse attempted to complete the follow-up call by the next business day. If the participant was unavailable, a voicemail message was left to call the nurse back. Readings that normalized before speaking with the participant resulted in no further action.
Statistical Considerations
Descriptive statistics were generated for patient demographics, treatment history, and outcome measures. The study was considered feasible if ≥80% of study participants performed a minimum of 3 weekly measurements using all Bluetooth-enabled devices each week. Acceptability of the RPM platform was assessed using a patient satisfaction survey administered at the time of study completion. Patient satisfaction responses were categorized using a 5-point Likert scale as agree (strongly agree/agree), neutral, or disagree (strongly disagree, disagree).
RESULTS
Thirty consecutive survivors who met the study’s eligibility criteria were approached, of whom 20 (67%) agreed to participate. Of the 20 who enrolled, 2 withdrew shortly thereafter because of worsening psychosocial and medical status. Information on the 18 participants who enrolled on the study and completed at least 1 requested measurement is included in Table 1. The median age at enrollment was 66 years (range, 53 to 74 years); 61% were female, 72% were non-Hispanic white, 44% had at least a college education, and 39% had undergone autologous HCT. The most common indication for HCT was acute leukemia (56%). The prevalence of hypertension, diabetes, and obesity was 67%, 22%, and 11% respectively; 44% of the HCT survivors were classified as high risk and 56% were classified as intermediate risk for CVD.
Table 1. Demographic and Clinical Characteristics of the Study Participants
Characteristic | Allogeneic (N = 11) | Autologous (N = 7) | Total (N = 18) |
---|---|---|---|
Sex, n (%) | |||
Male | 4 (36) | 3 (43) | 7 (39) |
Female | 7 (64) | 4 (57) | 11 (61) |
Ethnicity, n (%) | |||
White | 8 (73) | 5 (71) | 13 (72) |
Black/African American | 1 (9) | 1 (14) | 2 (11) |
Hispanic | 0 (0) | 1 (14) | 1 (6) |
Asian | 2 (18) | 0 (0) | 2 (11) |
Age, yr, median (range) | 67 (53-74) | 61 (53-72) | 66 (53-74) |
Diagnosis, n (%) | |||
Acute leukemia | 9 (82) | 1 (14) | 10 (56) |
Multiple myeloma | 0 (0) | 5 (71) | 5 (28) |
Other | 2 (18) | 1 (14) | 3 (17) |
Risk level, n (%) | |||
Intermediate | 6 (55) | 4 (57) | 10 (56) |
High | 5 (45) | 3 (43) | 8 (44) |
Education, n (%) | |||
<College | 5 (45) | 5 (71) | 10 (56) |
College | 6 (55) | 1 (14) | 7 (39) |
>College | 0 (0) | 1 (14) | 1 (6) |
Employment status, n (%) | |||
Employed | 3 (27) | 0 (0) | 3 (17) |
Unemployed | 2 (18) | 1 (14) | 3 (17) |
Retired | 6 (55) | 5 (71) | 11 (61) |
Declined to answer | 0 (0) | 1 (14) | 1 (6) |
Annual income, n (%) | |||
≤$49,999 | 6 (55) | 3 (43) | 9 (50) |
≥$50,000 | 4 (36) | 3 (43) | 7 (39) |
Declined to answer | 1 (9) | 1 (14) | 2 (11) |
Marital status, n (%) | |||
Never married | 3 (27) | 0 (0) | 3 (17) |
Currently married | 4 (36) | 4 (57) | 8 (44) |
Previously married | 4 (36) | 2 (29) | 6 (33) |
Declined to answer | 0 (0) | 1 (14) | 1 (6) |
Hypertension, n (%) | |||
Yes | 8 (73) | 4 (57) | 12 (67) |
No | 3 (27) | 3 (43) | 6 (33) |
Diabetes, n (%) | |||
Yes | 2 (18) | 2 (29) | 4 (22) |
No | 9 (82) | 5 (71) | 14 (78) |
Smoking history, n (%) | |||
Ever | 6 (55) | 3 (43) | 9 (50) |
Never | 5 (45) | 4 (57) | 9 (50) |
Obesity, n (%) | |||
Obese (body mass index ≥30 kg/m2) | 1 (9) | 1 (14) | 2 (11) |
Nonobese | 10 (91) | 6 (86) | 16 (89) |
As shown in Figure 2, there was an overall increase in the compliance rate from the first week to subsequent weeks, with 13 of 18 participants (72%) completing the requested measurements at least 3 times per week during the first week, and 15 out of 18 participants (83%) doing so in the subsequent 3 weeks; the mean weekly compliance rate for study participants was 80.6%. Of note, 12 participants (67%) successfully completed at least 3 readings per week using all devices for all 4 weeks. The BP monitor, scale, pulse oximeter, and glucometer recorded a total of 378, 373, 360, and 73 readings, respectively. We found differing values between clinic and home BP measurements obtained on the same day for the 9 participants who were seen in clinic during the study period. On average, systolic and diastolic values varied by ±9.2% and ±10.6% from their home values, respectively. Only 2 participants were able to successfully sync their Fitbit to the mTelehealth Patient Portal.

A total of 86 alerts were triggered throughout the study from 15 of the participants (83%). Four participants triggered 64% of all alerts. The majority of alerts (79%) occurred during the weekday when the nurse was providing active monitoring. Most of the readings (73%) normalized before the nurse’s phone call to the participant. BP readings triggered the most alerts (33%), and pulse oximetry the least alerts (1%). The study nurse made a total of 23 follow-up phone calls. All participants were either available when the nurse called or returned the call within 24 hours. Of the 23 calls, 15 (65%) resulted in a validation of the alert, a symptom check, and a request that the participant retake the reading. Additional reminders to take home medications were provided on 5 calls (22%). The remaining 3 calls required no action, because the alerts were accidentally triggered by individuals cleaning or stepping on the scale. The accuracy of the automatic alert system was evaluated by manual comparison of all entered values with the system thresholds. There were no false-positive or missing alerts.
Acceptability of the mTelehealth RPM Kit was evaluated using a 9-question Likert scale survey to evaluate the value of and satisfaction with the platform after 4 weeks of use. Participants had an overwhelmingly positive experience. All were glad that they participated in the study and felt comfortable using the devices. More than 90% of participants reported that the study kept them motivated and involved in their healthcare, and >85% agreed that the study supported their healthcare goals, helped them learn and manage their health conditions, and increased their access to healthcare.
DISCUSSION
The principal findings from the present study show that the mTelehealth RPM Kit is a feasible and highly acceptable platform with which to monitor modifiable risk factors in HCT survivors at risk for CVD. The mean weekly compliance rate was ≥80%, and at least two-thirds of the participants successfully performed ≥3 readings per week using all devices for 4 consecutive weeks. In fact, the majority of participants provided more than the requested readings, resulting in some compliance rates theoretically above 100%. The BP monitor was used slightly more than other devices. Considering that 67% of the participants had hypertension, the BP data might have been of increased interest to the study population. A major challenge in previous telehealth studies is a “honeymoon period” of high use, followed by a steep dropoff [13]. Even though our study lasted only 1 month, we were still surprised to find no dropoff. Moreover, the compliance rate increased after the first week. This may be explained by an initial learning curve followed by increasing confidence and motivation and greater levels of self-efficacy. Self-efficacy, a confidence in the ability to perform specific tasks or behaviors, which in turn influences the willingness to perform them, is considered an important driver of sustained behavioral change [11]. This may be especially relevant to our older population (age 53 to 74 years), who might not be as technologically proficient as a younger cohort.
More than 85% of participants agreed that the study allowed them to be more active in managing their health and kept them motivated and involved in their health care. According to the nurse’s log, 1 participant agreed to bring his study blood glucose readings to his physician, and another participant confirmed that he would find a dietician after consistently elevated blood glucose readings. All participants were glad they participated in the study and felt comfortable taking their own readings. It is important to note that for the present study, a nurse reviewed all patient-generated data and followed up on any readings that exceeded established thresholds. This “feedback loop” between a patient and a health care provider has been shown to improve the health status in chronically ill individuals [14]. It reinforces key messages and can encourage follow-up actions. For instance, the study nurse answered dietary concerns for 1 participant and encouraged him to meet with a dietician. Another participant was reminded to rest before taking her BP, teaching the importance of proper BP technique. Multiple participants were asked to take their home medications and then remeasure their BP, reinforcing the link between medication adherence and improved BP. Ideally, participants can then make these necessary adjustments independently in the future. As individuals become increasingly engaged in their care, the patient-provider relationship can evolve into one of improved collaboration.
It is noteworthy that the majority of alerts generated by the RPM platform were not actionable. That said, there was minimal alert burden on the monitoring provider, given that 73% of the alerts self-corrected and only 27% required follow-up calls by the study management and nursing team. Certain participants triggered regular alerts because of preestablished study thresholds; for example, 3 of the 4 participants with diabetes were on corticosteroid therapy. Their blood glucose levels were outside the fixed threshold but were appropriate for their current treatment. Another participant had weight fluctuations that were nonpathological. For this study, the thresholds were based on best practice values; future efforts would benefit from individualization of these thresholds. Interestingly, there was marked discrepancy in all 9 patients who had home and clinic BP readings on the same day, with the clinic readings often higher than the home average readings. It is widely recognized that clinic BP measurements can be misleading in a considerable portion of individuals, owing to a phenomenon known as “white coat hypertension,” in which BP can be elevated in the clinical setting, or to an opposite effect, known as “masked hypertension” 15, 16, 17, 18. Therefore, out-of-clinic BP monitoring may therefore be necessary to provide accurate information on steady-state BP readings [19]. Home BP monitoring has been shown to lead to better BP control, better compliance with antihypertensive therapy [20], and improved health-seeking behaviors 21, 22, 23. Since clinic time provides only a brief snapshot of the HCT survivors’ health care journey, longitudinal data from their home environment may provide a more comprehensive and holistic perspective. The current RPM strategy allows for more accurate projections of survivors’ physiological health, setting the stage for more comprehensive management of modifiable risk factors.
The primary limitations of our study are its small sample size and short study duration, which may limit the generalizability of our findings. A further limitation is that our findings are specific to the mTelehealth RPM platform and might not apply to all RPM technology. Importantly, home-based monitoring may need to be routinely validated with in-person clinic assessments, allowing healthcare providers to reinforce proper measurement techniques and to limit the likelihood of erroneous remote readings over time. Finally, it is important to note that RPM entails upfront costs and might not be feasible for all clinical settings in which HCT survivors are routinely seen. That said, these initial costs may be offset by the reduction of in-person clinic visits and complications associated with these risk factors, including CVD. Additional studies are needed to evaluate the cost-effectiveness and impact on patient outcomes using RPM and telemedicine in this population.
In conclusion, this study provides important information regarding the feasibility and acceptability of implementing a telehealth platform to monitor CV risk factors in HCT survivors at risk for CVD. Our preliminary findings may facilitate the development of population-based CVD interventions in large cohorts of survivors at a fraction of the cost and resources necessary to deliver preventive and long-term survivorship care. Importantly, it may set the stage for innovative delivery of care that encourages patients to be active participants in their health and reduces the burden of CVD in this growing population of patients. This paradigm of health care delivery could also be applied in acute care settings (eg, postoperative, outpatient HCT) across a range of scenarios for patients undergoing HCT and in long-term survivors.
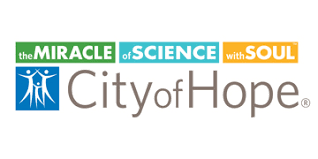
ACKNOWLEDGMENTS
The authors thank all those who participated in this study.
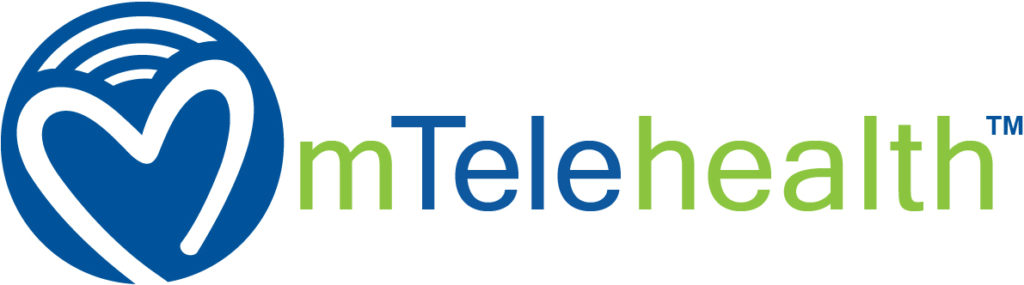
Financial disclosure: This research was supported by a Leukemia and Lymphoma Society Scholar Award in Clinical Research (2315-17; PI, S. H. Armenian), and through a seed grant from the Chapek family.
Conflict of interest statement: There are no conflicts of interest to report.
Appendix. Supplementary materials
REFERENCES
1Altekruse SF, Kosary CL, Krapcho M, et al, eds. National Cancer Institute. SEER cancer statistics review, 1975-2007. Available at: http://seer.cancer.gov/csr/1975_2007. Accessed January 14, 2020.Google Scholar
2NS Majhail, L Tao, C Bredeson, et al.Prevalence of hematopoietic cell transplant survivors in the United StatesBiol Blood Marrow Transplant, 19 (2013), pp. 1498-1501ArticleDownload PDFView Record in ScopusGoogle Scholar
3SH Armenian, S. BhatiaCardiovascular disease after hematopoietic cell transplantation—lessons learnedHaematologica, 93 (2008), pp. 1132-1136CrossRefView Record in ScopusGoogle Scholar
4SH Armenian, EJ. ChowCardiovascular disease in survivors of hematopoietic cell transplantationCancer, 120 (2014), pp. 469-479CrossRefView Record in ScopusGoogle Scholar
5SH Armenian, D Yang, JB Teh, et al.Prediction of cardiovascular disease among hematopoietic cell transplantation survivorsBlood Adv, 2 (2018), pp. 1756-1764CrossRefView Record in ScopusGoogle Scholar
6EJ Chow, BA Mueller, KS Baker, et al.Cardiovascular hospitalizations and mortality among recipients of hematopoietic stem cell transplantationAnn Intern Med, 155 (2011), pp. 21-32CrossRefGoogle Scholar
7PA. GanzA teachable moment for oncologists: cancer survivors, 10 million strong and growing!J Clin Oncol, 23 (2005), pp. 5458-5460View Record in ScopusGoogle Scholar
8SM Shankar, A Carter, CL Sun, et al.Health care utilization by adult long-term survivors of hematopoietic cell transplant: report from the Bone Marrow Transplant Survivor StudyCancer Epidemiol Biomarkers Prev, 16 (2007), pp. 834-839CrossRefView Record in ScopusGoogle Scholar
9H Jimison, P Gorman, S Woods, et al.Barriers and drivers of health information technology use for the elderly, chronically ill, and underservedEvid Rep Technol Assess (Full Rep) (2008;(175)), pp. 1-1422Google Scholar
10J Finkelstein, A Knight, S Marinopoulos, et al.Enabling patient-centered care through health information technologyEvid Rep Technol Assess (Full Rep) (2012;(206)), pp. 1-1531Google Scholar
11S Parker, A Prince, L Thomas, et al.Electronic, mobile and telehealth tools for vulnerable patients with chronic disease: a systematic review and realist synthesisBMJ Open, 8 (2018), Article e019192CrossRefGoogle Scholar
12M. GeorgeffDigital technologies and chronic disease managementAust Fam Physician, 43 (2014), pp. 842-846View Record in ScopusGoogle Scholar
13I. SimMobile devices and healthN Engl J Med, 381 (2019), pp. 956-968CrossRefView Record in ScopusGoogle Scholar
14PM Gee, DA Greenwood, DA PaternitiThe eHealth Enhanced Chronic Care Model: a theory derivation approachJ Med Internet Res, 17 (2015), p. e86CrossRefView Record in ScopusGoogle Scholar
15K Kario, TG Pickering, Y Umeda, et al.Morning surge in blood pressure as a predictor of silent and clinical cerebrovascular disease in elderly hypertensives: a prospective studyCirculation, 107 (2003), pp. 1401-1406View Record in ScopusGoogle Scholar
16TG Pickering, D Shimbo, D. HaasAmbulatory blood-pressure monitoringN Engl J Med, 354 (2006), pp. 2368-2374CrossRefGoogle Scholar
17Y Yano, K. KarioNocturnal blood pressure and cardiovascular disease: a review of recent advancesHypertens Res, 35 (2012), pp. 695-701CrossRefView Record in ScopusGoogle Scholar
18D Shimbo, M Abdalla, L FalzonRole of ambulatory and home blood pressure monitoring in clinical practice: a narrative reviewAnn Intern Med, 163 (2015), pp. 691-700CrossRefView Record in ScopusGoogle Scholar
19E O’Brien, R Asmar, L Beilin, et al.European Society of Hypertension recommendations for conventional, ambulatory and home blood pressure measurementJ Hypertens, 21 (2003), pp. 821-848View Record in ScopusGoogle Scholar
20BR Fletcher, J Hartmann-Boyce, L HintonThe effect of self-monitoring of blood pressure on medication adherence and lifestyle factors: a systematic review and meta-analysisAm J Hypertens, 28 (2015), pp. 1209-1221CrossRefView Record in ScopusGoogle Scholar
21RJ McManus, P Glasziou, A Hayen, et al.Blood pressure self monitoring: questions and answers from a national conferenceBMJ, 337 (2008), p. a2732CrossRefGoogle Scholar
22A Abdullah, S. OthmanThe influence of self-owned home blood pressure monitoring (HBPM) on primary care patients with hypertension: a qualitative studyBMC Fam Pract, 12 (2011), p. 143CrossRefView Record in ScopusGoogle Scholar
23BB Green, AJ Cook, JD Ralston, et al.Effectiveness of home blood pressure monitoring, Web communication, and pharmacist care on hypertension control: a randomized controlled trialJAMA, 299 (2008), pp. 2857-2867CrossRefView Record in ScopusGoogle Scholar